
We perform this technique for all three data splits. The final result is an interesting fusion of optical and SAR imagery.
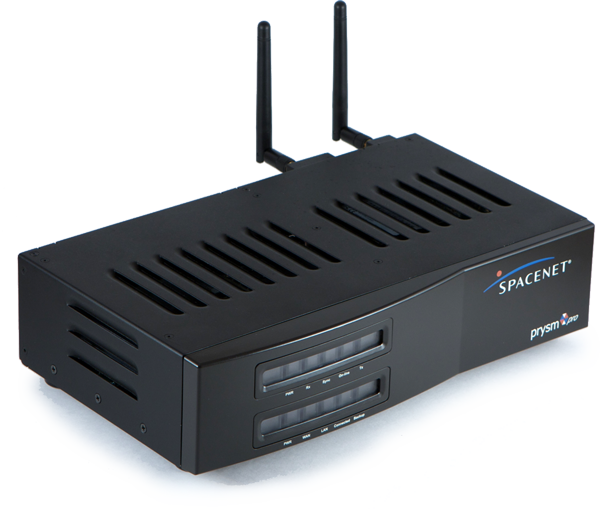

To achieve our analysis goals we constructed the following workflow: I.
#SPACENET MELBOURNE SERIES#
In this final blog in our post-SpaceNet 6 analysis challenge series (previous posts: ), we dive into a topic that we’ve been greatly interested in for a long while: Can colorizing synthetic aperture radar data with a deep learning network really help to improve you ability to detect buildings? The Approach and Results Moreover, we wanted to test this approach in an area where we didn’t have concurrent SAR and optical collects, simulating a real world scenario where this would commonly occur due to inconsistent orbits or cloud cover that would render optical sensors useless. We hypothesized that a segmentation network should find this color valuable to use for building detection. Will this help us improve the quality of our segmentation networks for extracting building footprints?Īfter seeing the image above, it triggered something in my mind - Instead of doing the direct conversion of SAR to optical imagery, what if we could work somewhere in the middle, training a network to convert SAR to look like the fused product shown above? On the surface this task seems far easier than the direct conversion and something that could be beneficial in a workflow. The inception of this workflow: Can we teach a neural network to automatically fuse RGB and SAR data? - As shown in the middle image above. The approach was intriguing as it maintained the interesting and valuable structural components of SAR while introducing the color of optical imagery. He had merged the RGB optical imagery and SAR data using a Hue Saturation and Value data fusion approach. We concluded that translating SAR data to look nearly identical to optical data appeared to be quite challenging at such a high resolution and may not be a viable solution.Ī few weeks later, one of our colleagues Jason Brown from Capella Space sent us an interesting image he had created. We had tried some similar approaches with the SpaceNet 6 data but had found the results underwhelming and the model outputs incoherent. Interestingly, some research had been done in this field, with a few approaches successfully transforming SAR to look like optical imagery at coarser resolutions ( 1, 2, 3). We thought that this transformation would be helpful for improving our ability to detect buildings or other objects in SAR data. When we first created the SpaceNet 6 dataset we were quite interested in testing the ability to transform SAR data to look more like optical imagery. SpaceNet is run in collaboration by co-founder and managing partner, CosmiQ Works, co-founder and co-chair, Maxar Technologies, and our partners including Amazon Web Services (AWS), Capella Space, Topcoder, IEEE GRSS, the National Geospatial-Intelligence Agency and Planet.
#SPACENET MELBOURNE DOWNLOAD#
Once you’ve done that, simply run the command below to download the training dataset to your working directory (e.g.Preface: SpaceNet LLC is a nonprofit organization dedicated to accelerating open source, artificial intelligence applied research for geospatial applications, specifically foundational mapping (i.e., building footprint & road network detection). The SpaceNet data is freely available on AWS, and all you need is an AWS account and the AWS CLI installed and configured. There are a few steps required to run the algorithm, as detailed below. While the goal is akin to traditional video object tracking, the semi-static nature of building footprints and extremely small size of the objects introduces unique challenges. This algorithm is a multi-step process that refines a deep learning segmentation model prediction mask into building footprint polygons, and then matches building identifiers (i.e. To address this problem we propose the SpaceNet 7 Baseline algorithm. The goal of the SpaceNet 7 Challenge is to identify and track building footprints and unique identifiers through the multiple seasons and conditions of the dataset.
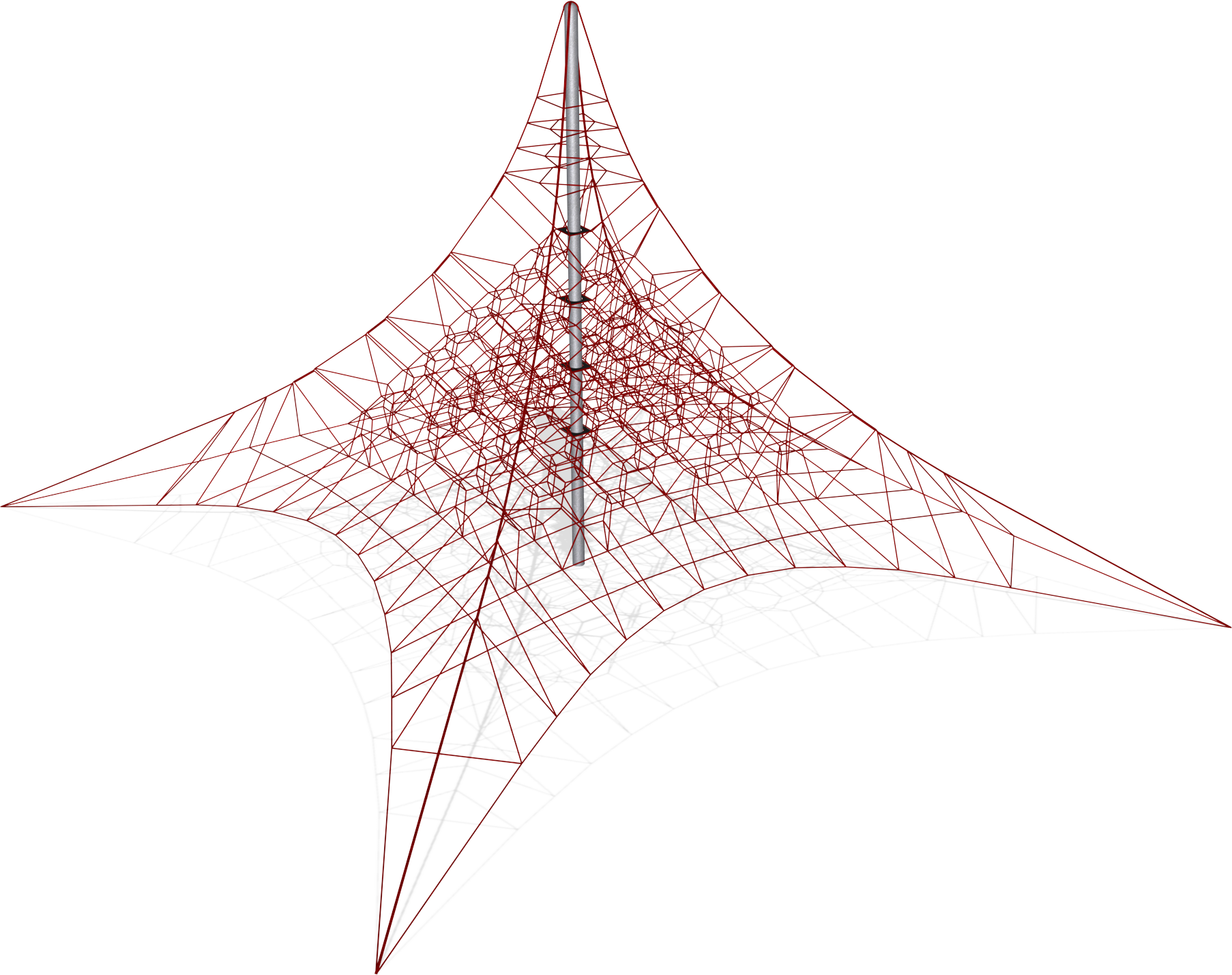
The SpaceNet 7 dataset contains ~100 data cubes of monthly Planet 4 meter resolution satellite imagery taken over a two year time span, with attendant building footprint labels.
